A broad and structured data investment
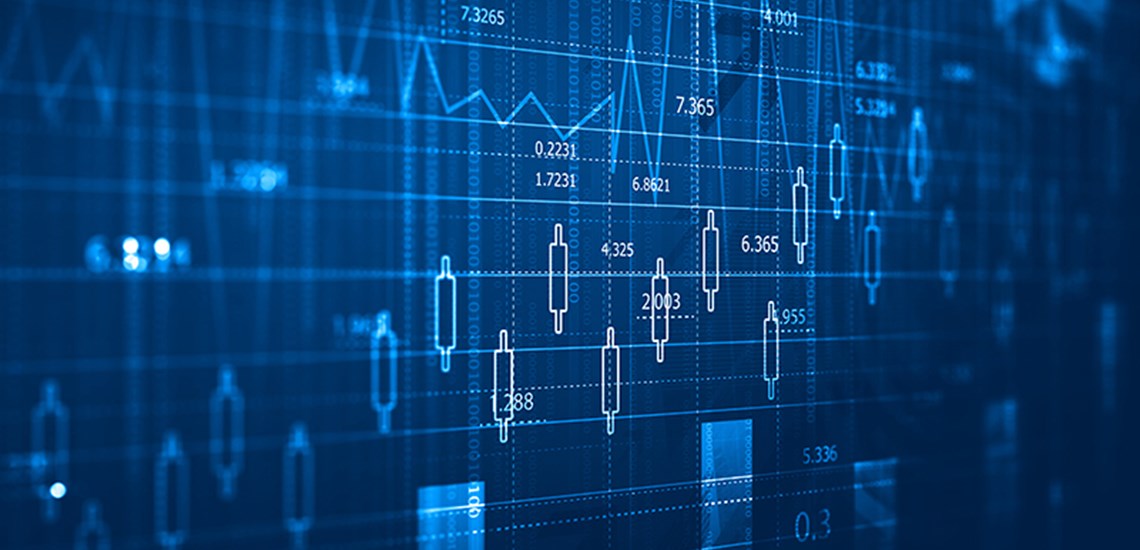
Let’s take a look at data stored through real-time data acquisition for your facility. How much live plant data do you keep? Do you apply business data retention policies to raw plant data ? Is this decision enforced by global decision makers and legal departments ? Does it seem like a sensible approach ?
In this post we will take a look at a few areas that demonstrate examples of decisions that should be driven from available facts if they are readily available. There are many others and we cannot know for sure what future technologies will be offering us. Technology moves forward at a startling rate and technologies like deep learning will almost certainly be more effectively and more commonly used in Manufacturing over the coming years. When these new capabilities are available to you will you have the information required to benefit from them immediately or will you need to start trying to gather and store new data at that point leaving yourself years behind your competitors.
Ok, the stage is set for a brief discussion on this topic. The only question we should ask is "What could my raw plant data retention policy cost me ?". Think about this briefly, is that figure positive or negative ?
Areas for improvement
Now, let's get this out of the way quickly and throw in a buzz word. Big Data. Anybody still there ? ...
Your data investment will without doubt reap rewards in the future. The nature of that return depends heavily on a number of factors, how much data have you got relating to your assets ?
Your answer might disguise the problem and convince you and your colleagues that you have more than enough. Is your answer in Terrabytes or in years ? Neither of those are particularly useful or meaningful when solving a puzzle. |
![]() |
Existing Data analysis
Let’s spread that data out over your facility to produce a heatmap. Some areas will be extremely data rich and other more tricky locations will come up short. Decisions will have been made at commissioning time that have never been reviewed since. There may be new I/O possibilities at these locations, some were available at the time but a little tedious to achieve. But hang on a minute, our heat map is useful but it is still a little one dimensional. This is only one layer in the heat map. We have built up the real-time data collection layer, what about routine maintenance, incidents and failures ? What about Supplier information, cost and MTBF analysis ?
Product quality implications impacted from other areas. Many of these are further complicated by improvements that are recognising less benefit than they should.
What data do we have and where is it ?
Automated real-time data collection from the facility might be an easy one to answer. That’s in our Pi, IP21 or Honeywell system. How much of this should we keep ? All of it, not necessarily all available on the live production system but why not? If pattern recognition tools improve or become more accessible to your engineers, they could discover cause and effect scenarios that were never previously considered.
Shift logs and maintenance records may start to get a bit more tricky. What about Customer feedback or Process Unit availability ? Is all of this data stored and is it readily available if needed. Some of these may be legacy systems that are considered in facility improvement plans already. It might be worth considering a more all encompassing Big Data solution.
Be Ready!
Ease of access to data that will speed up manual analysis is cost saving immediately. When a fully automated Deep Learning engine is ready to start analysing and providing us with valuable improvements it would seem sensible to be prepared and already have that wide angled lens view of all required data-sets. Don’t wait until it is ready, to realise that you are not.